AI Governance for Trustable AI -Part 1
with Koo Ping Shung, Practicum Director at Data Science Rex
This conversation with data scientist Koo Ping Shung is broken into two parts. In part one, we explore the topic of AI governance and how to build trustable AI. Koo discusses the evolution of the data science industry and the increasing focus on AI governance. He emphasizes that bias in AI cannot be completely eliminated but can be reduced through awareness and transparency. Koo suggests that AI governance committees should include individuals with technical, legal, policy-making, economics, and audit backgrounds. He also highlights the importance of AI literacy for consumers and the need for dedicated channels to address concerns and appeals.
See more from Koo Ping Shung
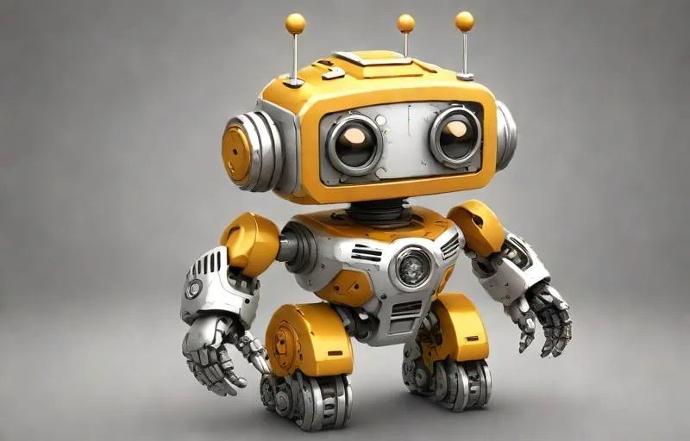
AI Ethics? AI Governance? Trust!
Sorry, after a few years of trumpeting the term "AI Ethics" and many (self-acclaimed) 'experts' on LinkedIn, I still do not believe in creating Ethical AIs. How so?
Working on AI Professional Ethics, is more intrinsic, whereas AI Governance is more extrinsic to get to AI that can be used.
What we really want to build at the end of the day is TRUSTABLE AI.
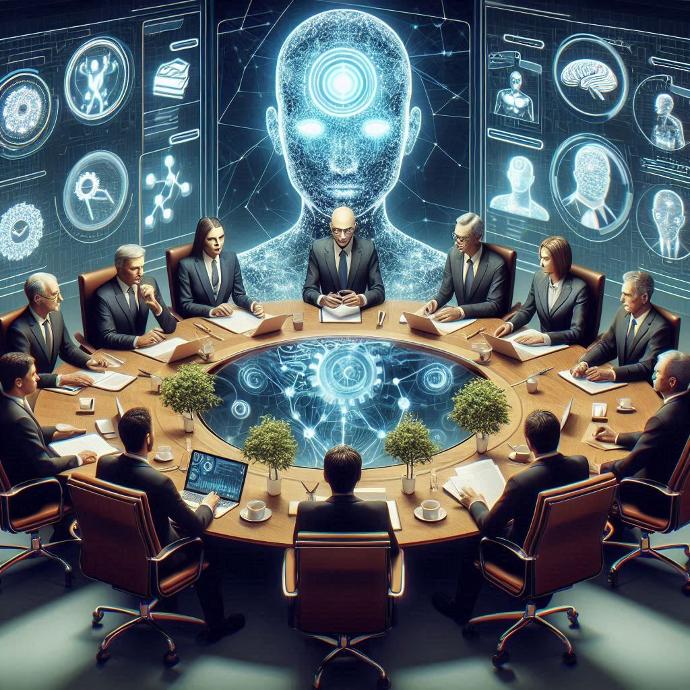
What Areas Should An AI Governance Committee Consist?
I want to shine the spotlight on AI Governance. Many organizations in recently years have organized their own AI Governance Committee. The reason is because the set up of these committees sends a strong signal that the organization is focusing on building Responsible AI, which is another buzzword these days.
https://koopingshung.com/blog/what-areas-should-an-ai-governance-committee-consist/
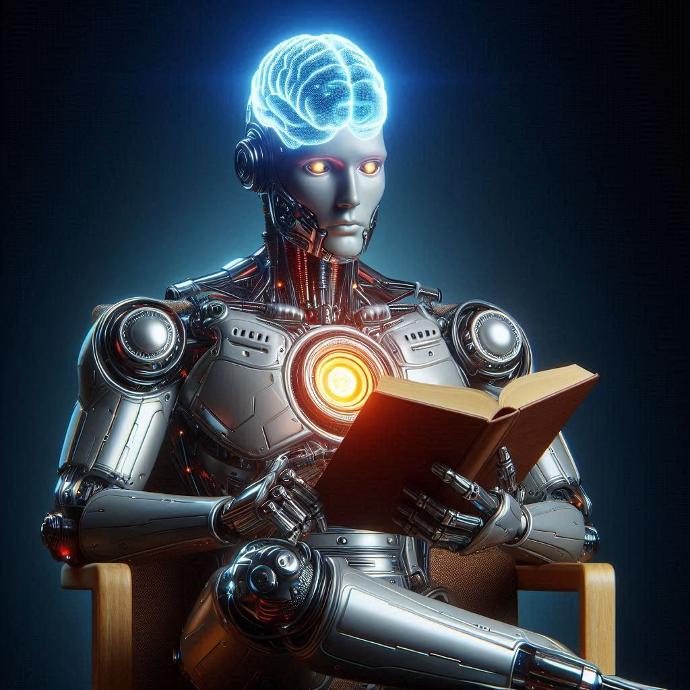
How Do We Test for Artificial General Intelligence (AGI)?
I'll be upfront, this post has a lot of questions more than my own answers to it and I am hoping I can reach out to more similar interest folks to have a good discussion from it. :)
https://koopingshung.com/blog/how-do-we-test-for-artificial-general-intelligence-agi/
Governance Complexity
AI governance is challenging due to the need for technical, legal, economic, policy-making, and regulatory expertise to prevent abuse and ensure effective use.
Bias in AI
Bias in AI is inevitable because data comes from humans with inherent preferences. The goal is to minimize bias as much as possible.
Transparency and Review
Effective AI governance requires transparency in decision-making processes and mechanisms to address biases and errors, ensuring disadvantaged groups can voice concerns.
Interdisciplinary Approach
Governance committees should include diverse skill sets, including technical, legal, economic, policy-making, and regulatory backgrounds, to address various aspects of AI use.
AI Literacy
Everyone should develop a basic understanding of AI to protect themselves from potential abuses and make informed decisions about AI's impact on their lives.
Trust in AI
Building trust in AI involves creating systems that are transparent, explainable, and capable of addressing biases and errors, ensuring AI benefits all stakeholders.
Jon Scheele (00:00)
Welcome to the API connections podcast. This conversation with data scientist Koo Ping Shung is broken into two parts. In part one, we discuss the complexities of AI governance and the need for expertise across several disciplines to make it effective.
We discuss bias in AI and the importance of transparency and a review mechanism to address that bias. And we discuss how it's up to all of us to gain AI literacy, so that we can ensure that AI works for us rather than against us. So enjoy part one.
Jon Scheele (00:35)
We've all been talking a lot about the opportunities, but also the dangers of AI.
For some people, the answer is better governance. But governance itself is a loaded term. It means different things to different people, and it's not actually easy to implement. So I'm very pleased to welcome Koo Ping Shung, the practicum director at Data Science Rex, to share his perspective on how we can build trustable AI. So welcome, Koo.
Koo Ping Shung (01:09)
Hey Jon, nice meeting you and thanks for the opportunity for me to share to your community on Trustable AI.
Jon Scheele (01:17)
Really pleased to have you and you've been in the data science game for quite some time. So you've seen some of the changes from the early use of analytics,
Jon Scheele (01:28)
predictive analytics, diagnostic analytics through to the current excitement about Large Language Models.
Concerns about things like bias and disinformation have been around even before large language models. And so there have already been some moves to address this at both government and corporate levels. Could you give sort of a picture of how you've seen the attempts at AI governance being shaped?
Koo Ping Shung (01:57)
So maybe let me just quickly share how the whole industry has evolved since I started working in this role. So what happened is I started my career in 2003. So that was during the SARS period. So unfortunately, whatever that's happening right now is a stark reminder of what happened when I started my career.
So back then, everything was about data.
The term that is usually associated with data is this term called Business Intelligence.
About visualization, reporting, trying to understand what's happening inside organizations. And the biggest user, of course, for business intelligence would be finance, banking, and all this.
Subsequently I went to this thing called Data Analytics, where now given that there's a lot more data, machine learning is now being used for the analysis work.
Data analytics exists for quite a fair bit, but it was quickly overtaken by another term called Data Science.
And before you know it, right, data science, although the term data scientist is still around, but most people's mind right now is actually on Artificial Intelligence which was, I think, brought about in 2016, if I'm, if my memory didn't fail me. Why 2016 was because of AlphaGo.
AlphaGo was a software that was created by DeepMind / Google. And it was playing the Go chess, which is considered the pinnacle of intelligence. And it's predicted that a machine won't be able to beat a human so soon.
And the timeline was actually 2035. That was what most researchers were saying that maybe by 2035, we can have a machine that can beat a human player in GoChess. And of course, it happened in 2016. So it was like 20 years earlier than predicted. And of course, that's where things start getting more, I would say, more interesting and more fun.
You start seeing AI in our smartphones. You start seeing AI in spam detection in our emails and stuff like that. And no thanks to Hollywood movies and other movies that you probably have seen, right? Then this is where people start to think, let their imagination run wild and say, you have to be very careful. AI can cause existential risk and so on and so forth. And this is where now in this particular year, especially with chat GPT right, bringing AI into a lot of people's mind. People start to talk about governance. We should govern our usage of I think that's the landscape right now, looking at it from the past 20 years. And so every country, in fact, not only everyone alone, but every country now also has been thinking about, hey, we need to think about governance. How do we ensure that we are using AI effectively, efficiently and for the good of humanity and so on and so forth.
Jon Scheele (05:00)
Yeah, so there have been a number of problems highlighted even before you look at AI. Simply any algorithm,
Jon Scheele (05:10)
whether it's machine learning, large language models, anything, has an inherent bias because it's the bias of the creator or the data that it's using to process.
And so when we look at the problems that that introduces, whether it may be because through racial discrimination, because most of the data is, or gender discrimination, because most of the data is based on white men in Western countries and doesn't necessarily apply to the rest of the world. And that has implications for whether people can get loans, or the sort of medical treatment that they should get.
So there's a whole range of problems with bias in the data, data sets themselves, and then the algorithms that process them. And then the challenges of bad actors introducing data of their own in order to spread disinformation. So there have been a number of initiatives, government initiatives. The Singapore government has one that they presented at the World Economic Forum. There are other countries have also introduced things.
But within companies who are planning to use things, there is also a need for governance to ensure that we're making good decisions, that are not disadvantaging individuals. So we want to do good business overall, but we also want to protect individual customers or stakeholders from the effects of a bad decision that we may make.
But the traditional way of doing governance in organizations, particularly large ones, is through committees that review things, that set standards and policies, and then look to see how they can enforce those. What's your view on how that's going to cope with this increased use of algorithms to make, not only present data to humans, but to make decisions often on their behalf?
Koo Ping Shung (07:49)
Okay, so a few things I want to address here. I think some of the things you pointed out like for instance, biasness and maybe we should even answer the question, what is governance in the first place? So at least my definition of governance will be to proliferate best practices and also to prevent abuse of AI being used of course in unfavorable situations. Maybe for instance,
For instance, like using it for weapons, for killing, for disadvantaging certain groups, and so on and so forth. So that would be how I term governance. But I wanted to address a bit more on the bias, on this thing about bias. I'm of the opinion that bias cannot be totally removed. There's no way you can surgically remove it so precisely that totally a data set doesn't have any bias. Why I'm saying that is because the data that we use comes from humans. And at the end of the day, we humans, let's not say we humans are biased, but we humans have our own preferences. And our preferences are built based upon what we have read, the kind of environment that we're brought up in, and also some of the maybe innate taste and flavor that you might actually have as well.
So a common example I always give to other people is when you go to a gelato shop. If you go to a gelato shop, you will definitely zoom into a few flavors before you even, before you even, how to put it, you won't go in and say, all of it is your favorite flavors because if all of them are your favorite flavors, you don't have a favorite at all.
So once you go in, there are certain flavors that you would like to have on that particular day. It could be maybe pistachio, it could be mint, it could be Belgian chocolate, or it could be dark chocolate, and so on and so forth. So with that in mind, naturally, we humans will have preferences, and preferences becomes negative. When preferences become negative, it becomes biased, because it hurts someone. It portrays someone maybe not as it is, negatively portraying someone. So that becomes a bias itself. And we humans, like I said, as long as we humans have preferences, there's a very good chance that we will be biased. So in that case, we humans are the ones who are producing the data that will be used to train the machines.
So we can't run away from the fact that data will be biased. The only thing what we can do as maybe model trainers, as people who use AI or whatever, is to be aware of it and try to reduce it to the minimum as much as possible. Of course, certain situations will require certain technical skills to be able to do that. But I think that's not the focus for this video or podcast. But I just want to, like I said, just to share in terms of bias, there's no way we can totally remove it. We can only just reduce it to the bare minimum as much as possible. Now, coming to the governance side,
I think for governance to be able to prevent any abuse and so on and so forth and looking at it from two perspectives. One is from a business organization perspective and the other one is coming in from maybe a country perspective or a national level perspective. Personally, I feel that
You definitely will need someone technical, someone who's familiar with AI and algorithms and the training of the models in the committee. In the committee, that's for sure. But if you're going to use it at sort of at the national level, if you're going to use AI at a national level, then it requires a lot more other things as well. Because like, for instance, if you want to prevent abuse, this is where you have to enact policies. You have to enact rules, you have to enact laws perhaps, or maybe even maybe just guidelines if you don't want it to be too strict. So this is why you need other people as well. So what other kind of backgrounds you probably will need right?
You probably need someone who is familiar with the technology law or risk management law and also a bit on jurisprudence, why do we even have the law, and how does setting up these law guidelines or everything else impact society? So that's one. I probably also will say that you need someone with some economics background, because you're using AI as a tool for innovation. Then you also have to see, for instance, what can we do to increase innovation, or accelerate the innovation cycle from an economics perspective, but also try to reduce the risk of it in the first place. So that's one. Economics, of course you need some people with policy -making background. Because policy -making background, you need to look at many, many different areas when you do a policy. Because once you implement a policy, you definitely impact many, many different areas. It could be manpower, it could be education, it could be healthcare, it could be environment.
So you need someone very good or very familiar with policy making, how to go about ensuring that the governance framework that's being set up can encourage innovation, but at the same time manage risk of using AI. So policy making. But of course, all this framework, however, that you mentioned, if you don't have auditors, if you don't have regulators coming into the picture, to say, hey, whether is this easy to regulate? Is it easy to make the audit? How do we ensure that certain levels is met, and so on and so forth? So that's why I also feel that you need someone with some audit background or regulation background to join the committee as well to be able to, again, like I said, be able to enforce the framework that's being set up by the committee altogether.
So these are just initial thoughts and areas where I feel that AI governance committee should have. So if you just on what I mentioned could be at the national level, but if you bring it down to the organization level, business organization level, then I will feel you probably will need someone from the marketing slash PR folks as well so that they know how to go about if anything happens, if anything happens, some issues because of
Koo Ping Shung (14:32)
using AI or whatever, this is where the PR and marketing folks can come in the picture all together. So some thoughts.
Jon Scheele (14:38)
So you mentioned lots of different people with lots of different skills. And I guess to pick up on the first point that you made is that recognizing that we all have some bias. So recognizing requires not just recognizing, but you also want to be able to quantify what that bias is and also have some transparency about it. So
Jon Scheele (15:03)
One of the challenges of bias is that when we don't recognize it, we don't have or develop a mechanism for addressing some of the downsides of that bias. If we're going to disadvantage a certain group, then there should be a mechanism for somebody to say, well, it's not working in my case. The algorithm might work 99 % of the time. But in my case, it's not.
Jon Scheele (15:29)
It's making the wrong decision, it's disadvantaging me. And so having that review mechanism. But you can't have a review mechanism if you don't have the transparency of it. And if you don't know how that algorithm has made that decision. And particularly with the different skill sets that you talked about, you don't find all those skills in one person. You have to have people from different perspectives to bring together
Jon Scheele (15:58)
the legal, the policy, the regulatory, the audit, the marketing, all those sorts of skills. So it has to be explainable. And Einstein said something like, if you can't explain it to a five-year-old, then you don't really understand it. But this is one huge challenge of AI is that often even the data scientists who have created this model don't know exactly what the model is going to recommend in a given case because the neural network may follow a different path and come up with a slightly different recommendation. It may generate a different output. So what do you see as being important for all of these different people to understand?
Given that they're not going to become data scientists themselves, what would you recommend for specialists who are not data scientists to understand about the AI and what they should contribute to that sort of governance discussion?
Koo Ping Shung (17:13)
I think we're coming from the consumer perspective, whether they get abused or not, and I'll put it as whether it's a reasonable abuse in the first place. So this is where I think literacy comes in the picture. And I do feel that going forward, every one of us will have to be literate in data. We also will have to be literate in AI. So now, here's the thing. Literacy doesn't mean that you become a professional. Literacy means you understand how it works. You don't have to. So it's how to put it. You know how to drive the car, but you don't need to know how the car actually works. So that's what I meant by literacy and I think going forward again, I said everyone everyone would need to Have some level of literacy with regards to data and regards to AI now Why is that the case? Let's just look at AI literacy alone. So now once you once you are literate with AI you understand how how how AI works So first thing first is you have a very good idea how to protect yourself first How to protect yourself from being abused?
That's one. Second thing is also you will also have some idea whether you've been abused because you roughly have some idea. This certain expectation, certain expectation that I shouldn't be getting they have a very good idea. And when they raise a point, if it's valid, people will listen.
If it's not valid, and people will just say that, hey, you're just creating nonsense. It's something that we can't act upon. But if you're literate, you know what's possible and what's not possible. The way you convince, the way you get someone to hear from you, it's a lot more credible.
Jon Scheele (19:08)
You brought up the analogy of driving a car, because I often use that analogy when I'm describing the benefits of using APIs. Because pretty much, a lot of people know how to drive a car, but very few people know, if you lifted the bonnet, people might be able to identify that that's the engine there, and that's the battery, and here's where I checked the oil, but they don't know anything else.
Jon Scheele (19:36)
But the thing is they don't need to know because the complexity of the car is taken away from them It's hidden from them. It's under that bonnet and they interact with the car through its interfaces You have a handle to it so you can open the door, you sit behind the the wheel. The steering wheel is how you tell the car which direction you want to go.
Here the pedals on the floor describe how fast you want the car to go when you want it to stop. So these interfaces are what helps you interact with something that's so complex that you don't understand.
With AI, what are the interfaces that we can really use when you talk about, well, what do we expect from the inputs that we provide and the outputs that we get from it? How are we going to measure, am I getting the right result for me? Am I not being abused by the algorithm? Am I not being disadvantaged? Is it the right result for me? And if I need to raise the flag and say this isn't right, what are the sorts of things that I should be thinking about explaining to people?
Because if I call up a contact center of a major company and I say your algorithm doesn't work, or it hasn't done the right thing by me, I don't think I'm going to get a very helpful answer because the contact center agent isn't going to say, well, I'd probably just say, I don't understand the algorithm either, but why is the algorithm the problem? Maybe it's you.
Koo Ping Shung (21:33)
I mean...
I think the consumer should go in and say that it's the algorithm that has a problem because that itself is a bias already because you're already saying that the algorithm is the problem which may not be the case until we really do an investigation. However, you do touch on quite a good point in the sense that hey, how do we as consumers raise the risk so that there's a double check.
Koo Ping Shung (22:00)
Not necessarily that it's a problem, but at least there is an opportunity to raise it up and double check, make sure that you're not subjected to abuse or the biases came from someone else. I think this is where companies who wants to still earn the consumer dollars will have to work on it, especially, let's say, if they want to still continue to tap onto AI. Because again, going back, like I said, as long as we're going to use AI, there won't be biases. There will be biases. That's one. Second thing is also, if the trend right now continues where most people use machine learning to build AI tools,
Any company will then have to deal with false positive and false negative as long as you're using machine learning, especially supervised learning, which in that case, you have to have a feedback process or mechanism built. So what I mean by that would be, so maybe the company who wants to continue to use using AI, especially for consumer businesses and so on and so forth.
They should have a dedicated channel for people to raise issues, to raise maybe a second look on the decision. But if you put yourself in the shoe of the business, that's also the other thing about how do we prevent abuse. I mean, if we don't do that kind of prevention of abuse, what's going to happen is any time someone come across an unfavorable decision, he or she is just going to raise it regardless.
So definitely will appeal for it. So how do we go ahead and reduce that abuse also as well? I think that's also another challenge to think about, I'll say. But I think one day I will agree.
Jon Scheele (23:56)
And in the legal system, there are corollaries of that because there needs to be a mechanism for somebody to appeal a decision that has gone against them if they feel that they haven't had a fair trial. But then there also needs to be a mechanism on the other side to prevent
I think it's called vexatious litigation where somebody's hammering away saying you did the wrong thing when actually they are at fault and trying to get a better result simply by perseverance. But there have been cases where algorithms or decisions about technology have disadvantaged some people even without the machine learning aspect of it.
In Australia, people are aware of the robo debt problem where some algorithm decided that people had been given government benefits that they didn't deserve and they needed to pay it back. And these people didn't really feel that they had a mechanism for disputing that decision. And so there is...
Jon Scheele (25:20)
That feedback mechanism, in order to ensure that people don't get disadvantaged, there needs to be some way for them to raise that and be heard. But as you said, there are always people who are trying to game any system. So it needs to be that companies also need to recognize, need to have a good filtering mechanism for that feedback.
Because the feedback, making good decisions about that feedback also has its own Type I and Type II errors or false positive, false negative errors in it. So I guess that the challenge though is where does it stop and at what point do you say I need to escalate over the top of this whole process because this is not being resolved.
Jon Scheele (26:21)
I hope you enjoyed part one of my conversation with Koo. In part two, we explore the challenges organizations face with data practices and the impact this has on API governance. We talk about the need for documentation and knowledge management within the organization, and the role of AI itself in enhancing documentation efforts, and the need for continuous improvement.
Start with the customer – find out what they want and give it to them.
powered by blue connector
API Strategy and Tech Advisory, Training and Events
We connect your organisation, your customers, partners and suppliers with the information and knowledge you need to make your tech work for you